Abstract |
Over the past few years, deep learning (DL) algorithms have dramatically increased in popularity, especially in the field of remote sensing. Image segmentation basically involves the detection and classification of individual objects within the image. In case of satellite images, the objects may be buildings, roads, vegetation, waterbodies, and so on. In the present work, DeepLabv3+ which is a state-of-the-art network is used to extract water bodies. It has an encoder–decoder architecture with atrous convolution between encoder and decoder. Encoder is used to extract high-level information from the input image. This extracted information is then further used for the segmentation task. Quality of encoder architecture used therefore has significant impact on the result of segmentation task. The existing encoder architecture in DeepLabv3+ is Xception. Along with this, ResNet-50 and U-Net are the other two encoder architectures that are considered for this study. |
Authors |
S. Adarsh , V. Sowmya , Ramesh Sivanpillai 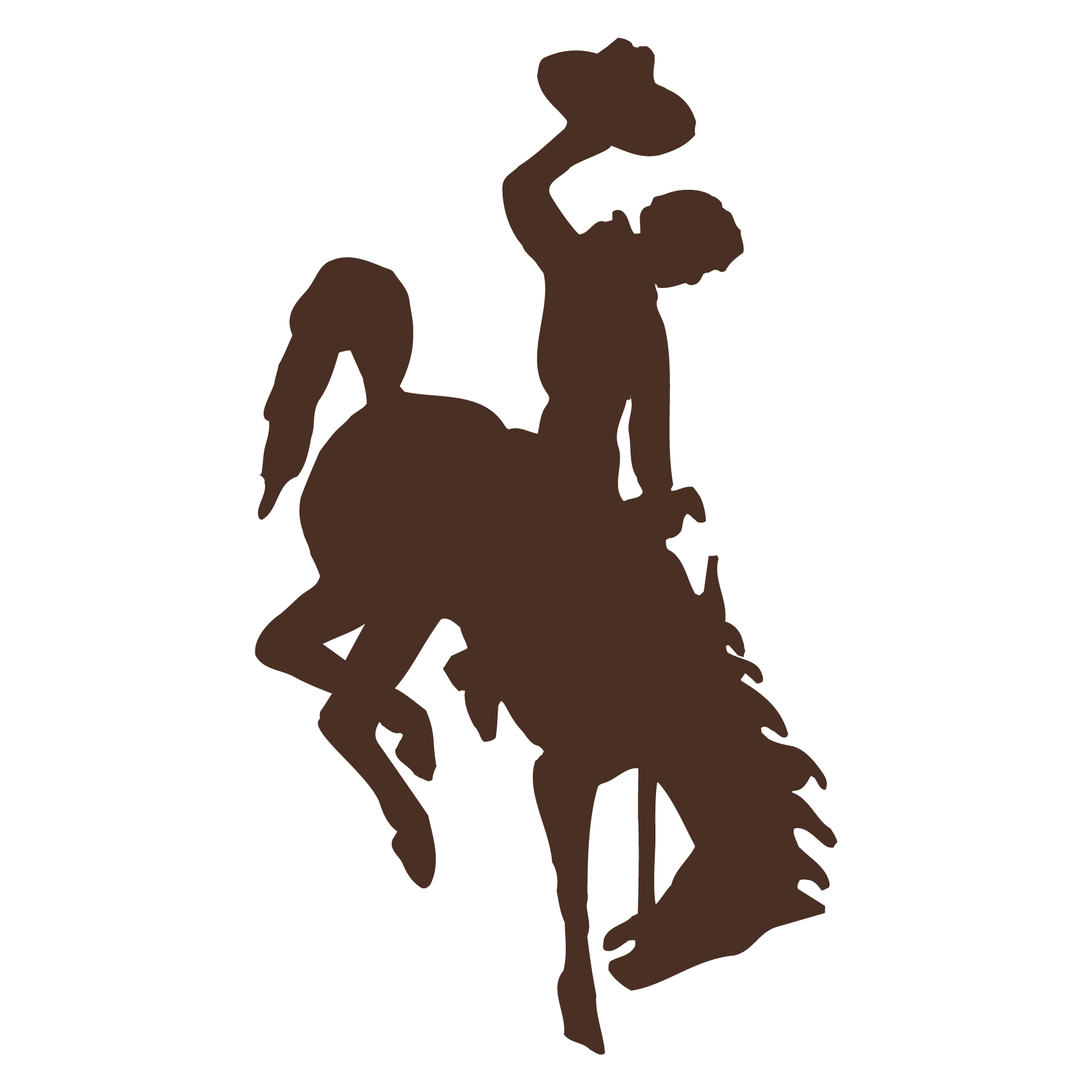 , V. V. Sajith Variyar
|
Journal Info |
Springer International Publishing | Inventive Communication and Computational Technologies , pages: 499 - 511
|
Publication Date |
10/4/2023 |
ISSN |
2367-3389 |
Type |
book-chapter |
Open Access |
closed
|
DOI |
https://doi.org/10.1007/978-981-99-5166-6_34 |
Keywords |
Deep Learning (Score: 0.539651) , Lidar Remote Sensing (Score: 0.517808) , High-Resolution Imagery (Score: 0.500404)
|