Abstract |
This study addresses the challenge of analyzing intensive longitudinal data (ILD) with zero-inflated autoregressive processes. ILD, characterized by intensive longitudinal measurements, often exhibit excessive zeros and temporal dependencies. Neglecting zero inflation or mishandling it can lead to biased parameter estimates and inaccurate conclusions. To overcome this issue, we propose a novel zero-inflated process change multilevel autoregressive (ZIP-CAR) model that incorporates zero inflation using a Bayesian framework. We compare the performance of the proposed method with existing methods through a simulation study and demonstrate its advantages in accurately estimating parameters and improving statistical power. Additionally, we apply the ZIP-CAR model to a real intensive longitudinal data set on problematic drinking behaviors, highlighting its effectiveness in capturing autoregressive and cross-lag effects while accounting for zero inflation. The results underscore the importance of addressing zero inflation in ILD analysis and provide practical recommendations for researchers. Our proposed model offers a valuable tool for analyzing ILD with zero-inflated autoregressive processes, facilitating a more comprehensive understanding of dynamic behavioral changes over time. (PsycInfo Database Record (c) 2025 APA, all rights reserved). |
Authors |
Sijing Shao , Ziqian Xu , Qimin Liu , Kenneth McClure 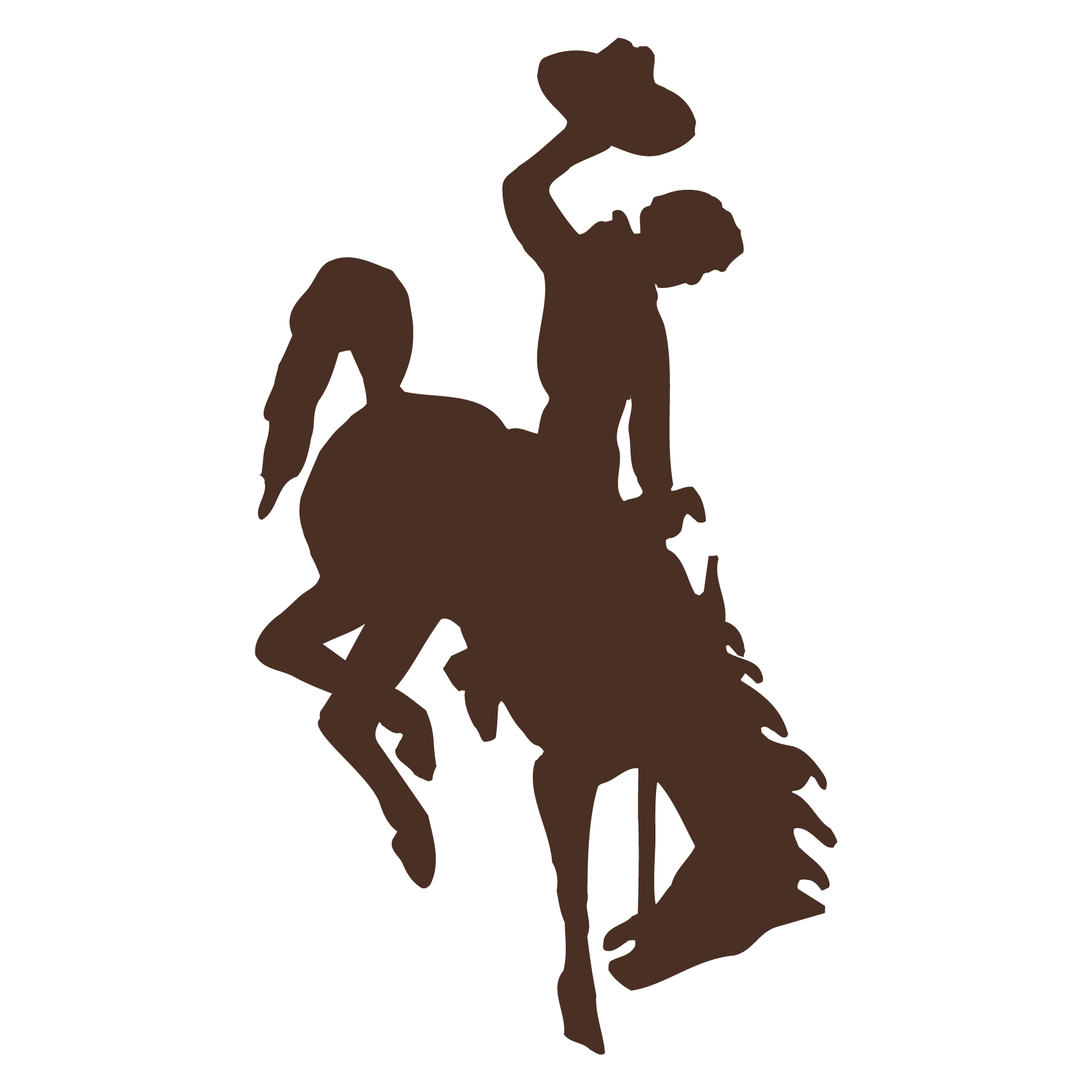 , Ross Jacobucci , Scott E. Maxwell , Zhiyong Zhang
|
Journal Info |
American Psychological Association | Psychological Methods
|
Publication Date |
4/7/2025 |
ISSN |
1082-989X |
Type |
article |
Open Access |
green
|
DOI |
https://doi.org/10.1037/met0000754 |
Keywords |
Zero (linguistics) (Score: 0.7136532) , Longitudinal data (Score: 0.5152757)
|