Abstract |
Malicious URLs are one of the most common and active vectors for launching cyber-attacks such as spam, phishing, social engineering, and malware. They result in billions of dollars in annual losses and call for effective detection techniques. Machine-learning-based detection is among the most promising candidates, but its performance depends on various factors, including the proper selection of representation features. This step often requires special expert domain knowledge and may be carried out manually, particularly in unique and specialized applications. This paper proposes an approach combining several methods (information gain, genetic algorithms, random forest) to select a small set of representation features to train the ML model efficiently. Experimental results show that ML models trained from the selected features yield comparable performance to the traditional approach while requiring less time and computational resources. |
Authors |
Sajjad Hussain Shah , Amit Garu , Duong Nguyen 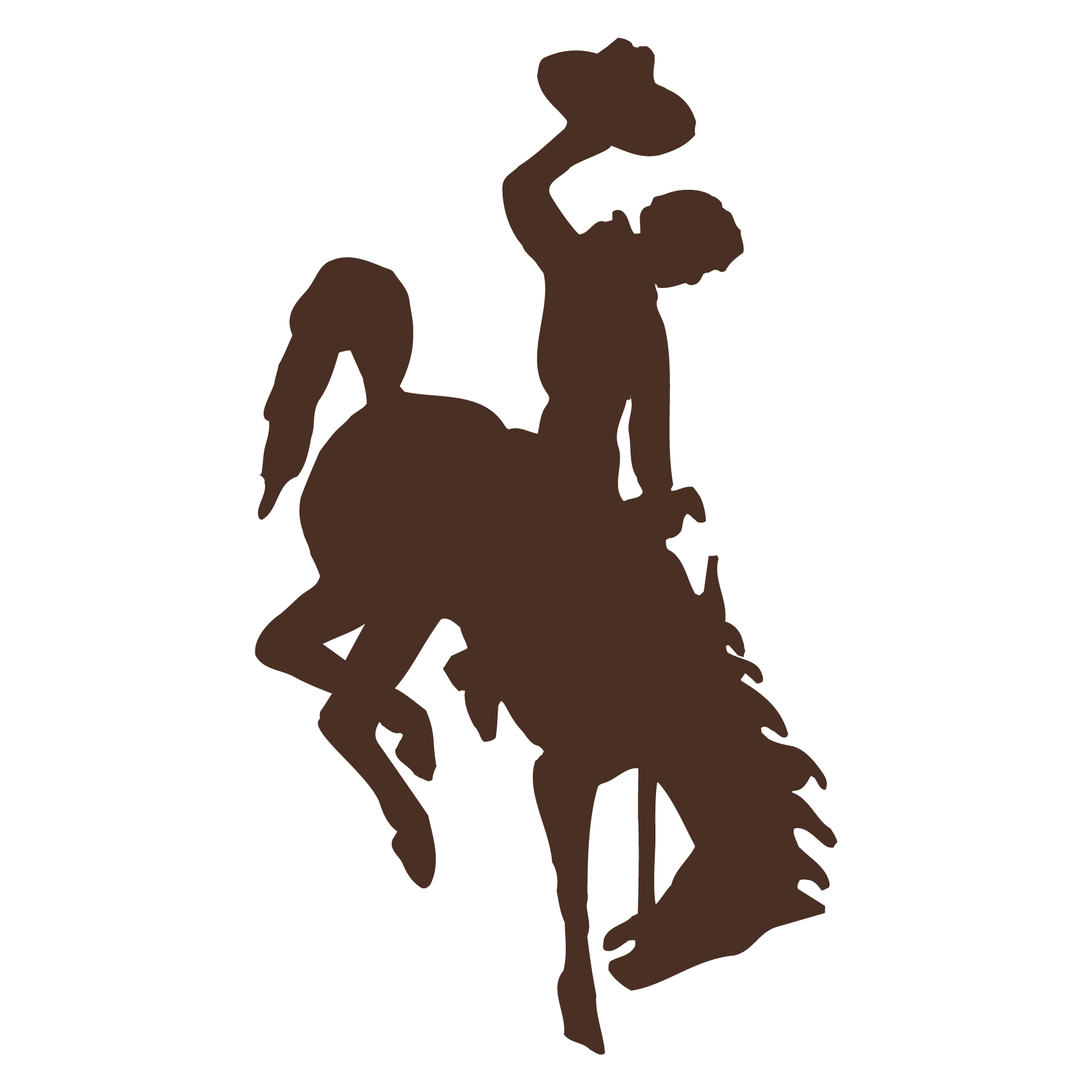 , Mike Borowczak
|
Journal Info |
Institute of Electrical and Electronics Engineers | Cyber Awareness and Research Symposium (CARS) , pages: 1 - 6
|
Publication Date |
10/28/2024 |
ISSN |
|
Type |
article |
Open Access |
closed
|
DOI |
https://doi.org/10.1109/cars61786.2024.10778786 |
Keywords |
Feature (linguistics) (Score: 0.56885695)
|