Abstract |
This paper presents an EEG-based user authentication system using Event-Related Potentials (ERPs) to distinguish legitimate users from impostors. Utilizing a publicly available EEG dataset, we implemented a comprehensive data processing pipeline, which included advanced preprocessing and feature extraction techniques. Multiple state-of-the-art machine learning classifiers, such as CatBoost and XGBoost, were evaluated to assess their effectiveness in user authentication. The results showed a very low average Equal Error Rate (EER) of 2.53%. Our study emphasizes the strength of the P300 and N400 responses in biometric authentication and demonstrates the potential of advanced ensemble classifiers in improving system accuracy. This research contributes to the development of EEG-based authentication and lays the groundwork for future studies aiming to create secure and practical biometric systems. |
Authors |
Soudabeh Bolouri , Diksha Shukla 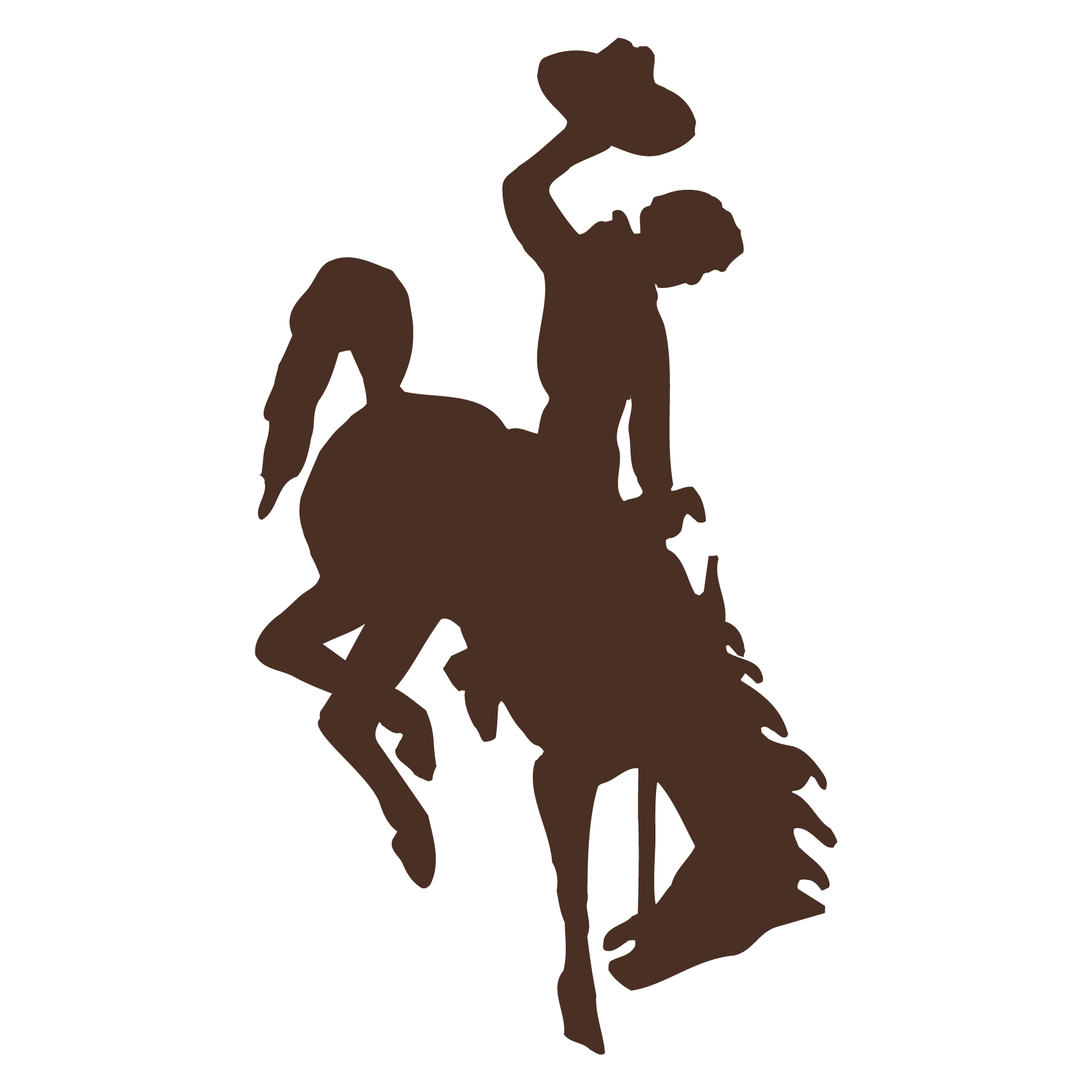
|
Journal Info |
Institute of Electrical and Electronics Engineers | 2024 Cyber Awareness and Research Symposium (CARS) , pages: 1 - 6
|
Publication Date |
10/28/2024 |
ISSN |
|
Type |
article |
Open Access |
closed
|
DOI |
https://doi.org/10.1109/cars61786.2024.10778891 |
Keywords |
Ensemble Learning (Score: 0.542398)
|