Abstract |
Sampling-based stochastic optimal control has become an appealing robotic control framework due to its ability to handle complex and general forms of dynamics models and task specifications. Although sampling-based methods have been shown successful in a variety of single-robot control tasks, studies on their extension to multi-robot problems are limited. In this letter, we propose a distributed framework for sampling-based optimal control. The framework formulates multi-robot optimal control as probabilistic inference over graphical models and leverages belief propagation to achieve inference via distributed computation. We developed a distributed sampling-based model predictive control (MPC) algorithm based on the proposed framework, which obtains optimal controls via variational inference. The algorithm was validated in a multi-robot formation navigation problem. The simulation results show the efficacy of our proposed method with improved control performance over a gradient-based distributed MPC algorithm. |
Authors |
Chao Jiang 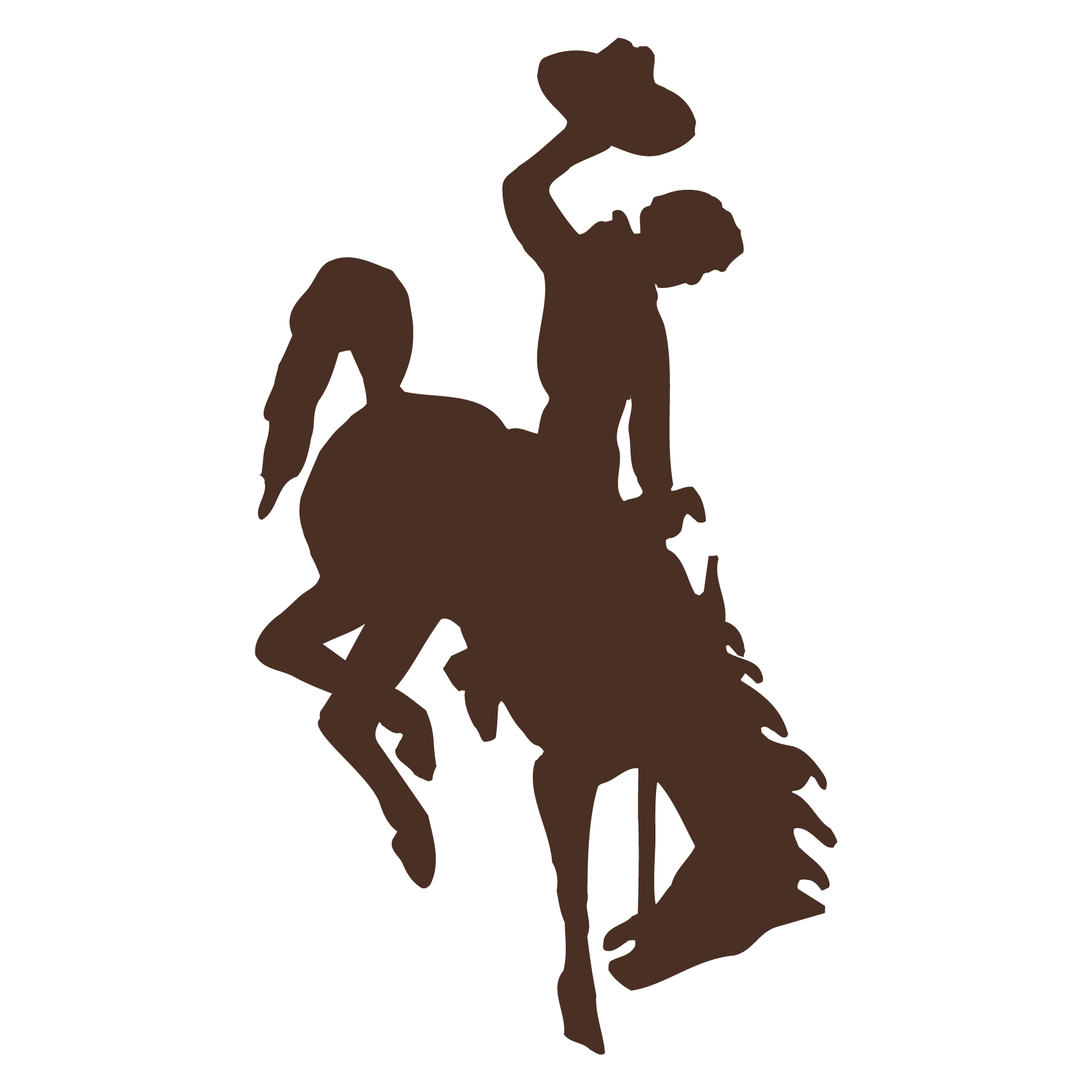
|
Journal Info |
Institute of Electrical and Electronics Engineers | IEEE Robotics and Automation Letters , vol: 9
, iss: 4
, pages: 3467 - 3474
|
Publication Date |
4/1/2024 |
ISSN |
2377-3766 |
Type |
article |
Open Access |
closed
|
DOI |
https://doi.org/10.1109/lra.2024.3368794 |
Keywords |
Model Predictive Control (Score: 0.63627) , Formation Control (Score: 0.622327) , Distributed Control (Score: 0.618078) , Distributed Optimization (Score: 0.607042) , Robust Control (Score: 0.582636)
|