Abstract |
Composite power system reliability assessment faces computational efficiency and accuracy challenges as systems expand with diverse generation resources. Conventional methods rely on optimizing load curtailment with power flow constraints for each sample state, which is computationally expensive. Machine learning techniques have emerged as a potential re-placement for such issues with two main approaches: classifying failure/success states and predicting load curtailment. The classification approach does not estimate the energy indices, and the regression approach incurs high errors while estimating probability indices. To overcome these drawbacks, this paper proposes an improved methodology integrating classification and regression techniques for composite reliability assessment. Solving the optimization for load curtailment with power flow constraints is utilized during training data generation. The classification model is trained to distinguish success and failure states. Subsequently, only the failure states are processed through a regression model to predict load curtailment. The results indicate that this proposed approach enhances the fit of the regression model and improves the accuracy of reliability indices. |
Authors |
Ram Prasad Pandey , Ujjwol Tamrakar , Nga Nguyen 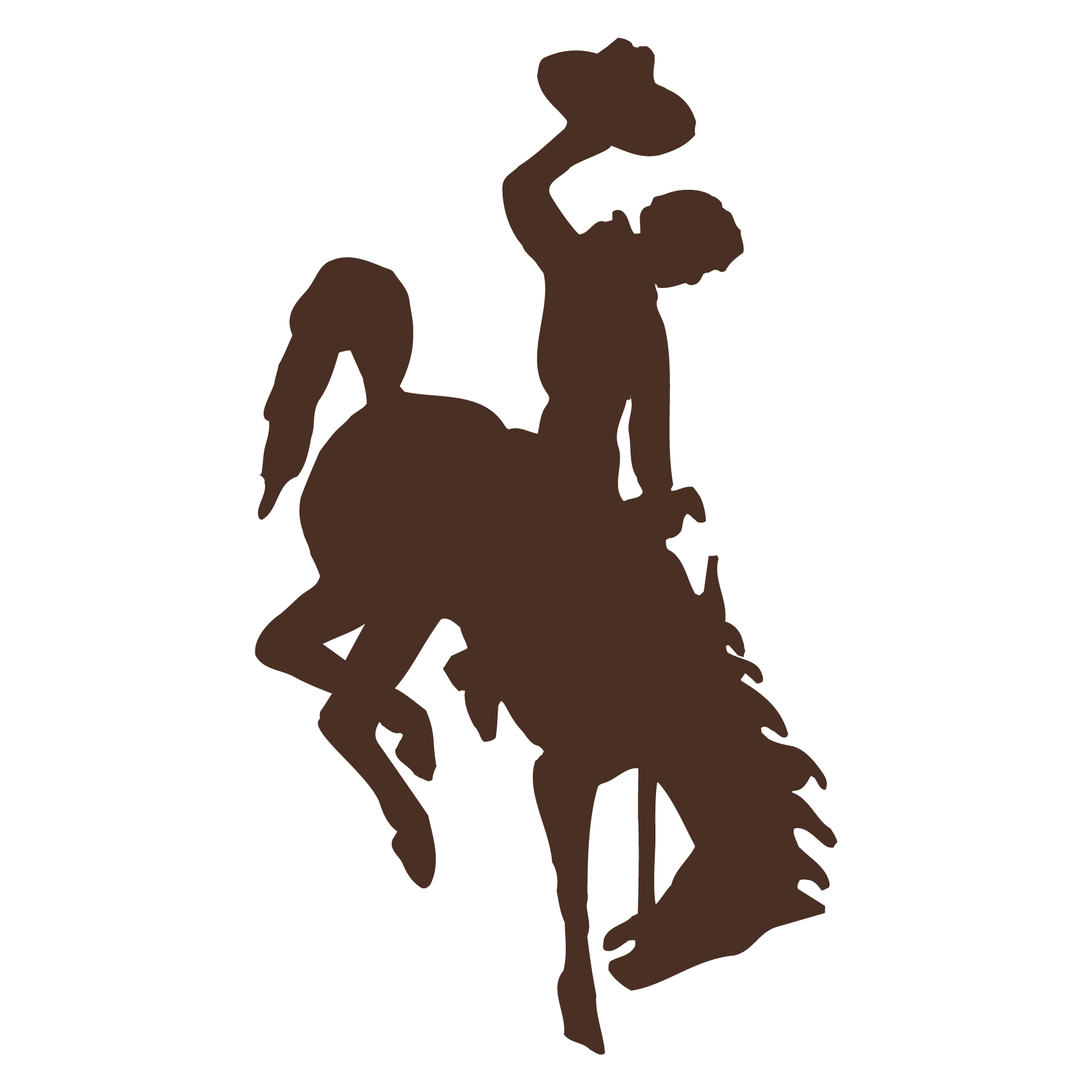
|
Journal Info |
Institute of Electrical and Electronics Engineers | 2024 56th North American Power Symposium (NAPS) , pages: 1 - 6
|
Publication Date |
10/13/2024 |
ISSN |
|
Type |
article |
Open Access |
closed
|
DOI |
https://doi.org/10.1109/naps61145.2024.10741696 |
Keywords |
|