Abstract |
The accuracy and computational feasibility of composite reliability assessment (CRA) have become a significant challenge with the increasing size of power grids and the penetration of highly intermittent renewable sources. Since the nonsequential Monte Carlo simulation (MCS) and analytical models cannot incorporate time-dependent quantities, improving the computation speed of sequential MCS for CRA is essential. This paper proposes a new method for CRA based on deep neural networks (DNN) within the sequential MCS framework. The DNN is designed to replace the computationally expensive optimal power flow solved in every time-step within the CRA. The DNN is trained as a regression algorithm that estimates the generator, energy storage dispatch, and load curtailment. A novel training strategy based on generator failure rates and load levels is implemented for efficient training of the DNN. The training and testing datasets are generated using the sequential Monte Carlo simulation, which considers generator ramping limits and energy storage operation. The IEEE RTS is utilized for testing and validating the proposed approach. |
Authors |
Dilip Pandit 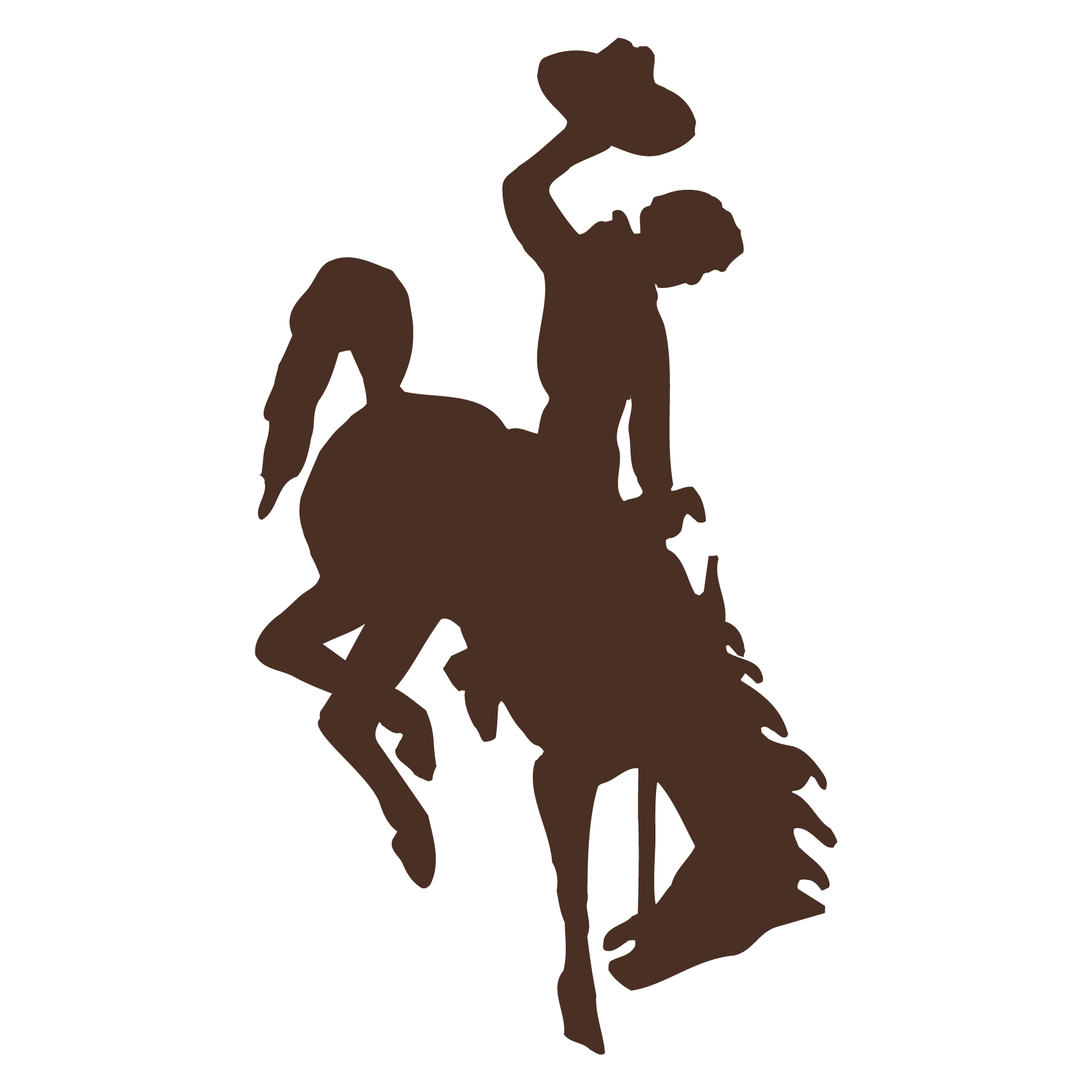 , Nga Nguyen 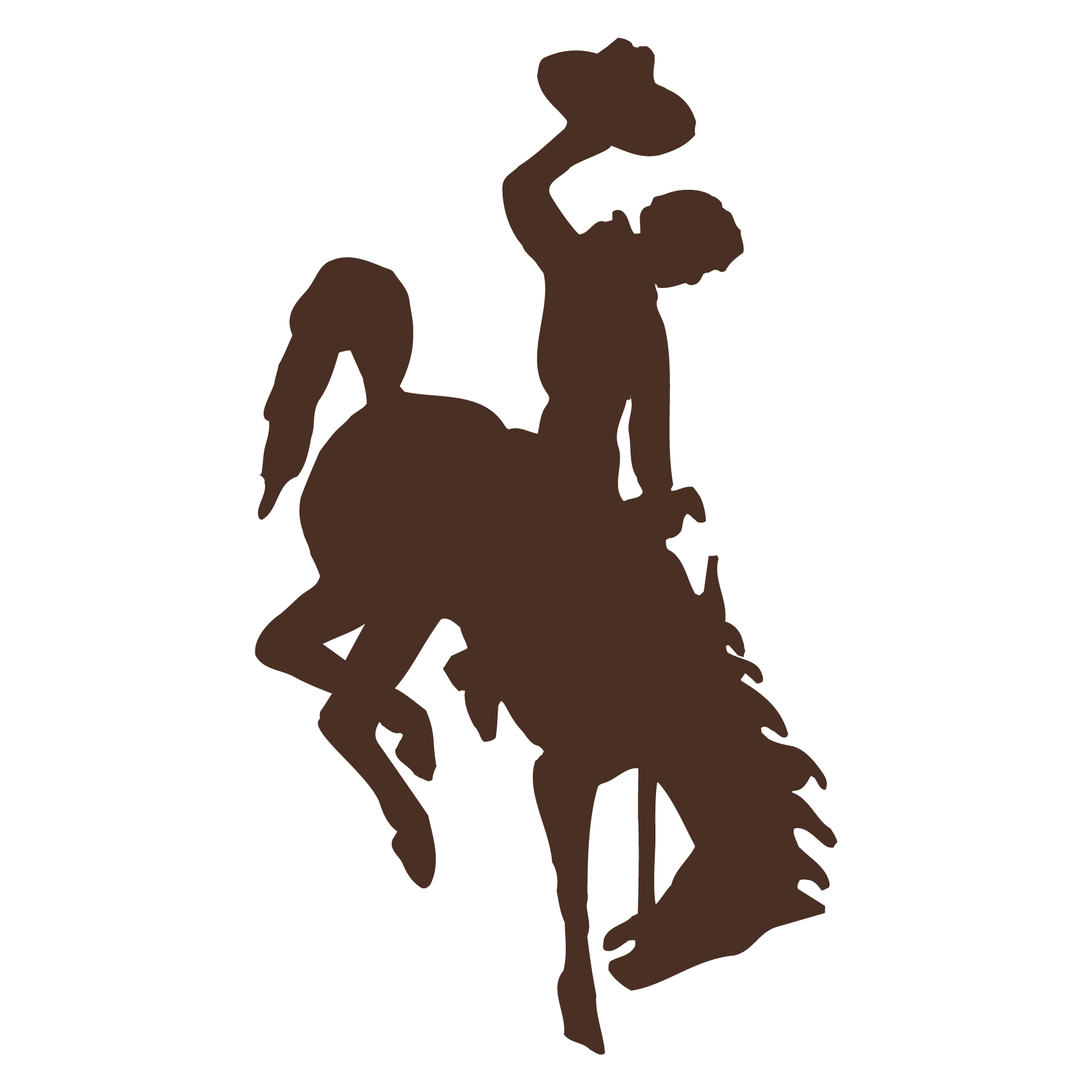
|
Journal Info |
Institute of Electrical and Electronics Engineers | 2023 IEEE Power & Energy Society General Meeting (PESGM)
|
Publication Date |
7/16/2023 |
ISSN |
Not listed |
Type |
article |
Open Access |
closed
|
DOI |
https://doi.org/10.1109/pesgm52003.2023.10252304 |
Keywords |
Composite Reliability (Score: 0.628679) , Reliability Evaluation (Score: 0.549273) , Dynamic State Estimation (Score: 0.519988) , Optimal Power Flow (Score: 0.515219) , Optimization (Score: 0.514737)
|