Abstract |
The U-Net-like coarse-to-fine network design is currently the dominant choice for dense prediction tasks. Although this design can often achieve competitive performance, it suffers from some inherent limitations, such as training error propagation from low to high resolution and the dependency on the deeper and heavier backbones. To design an effective network that performs better, we instead propose Recurrent Multiscale Feature Modulation (R-MSFM), a new lightweight network design for self-supervised monocular depth estimation. R-MSFM extracts per-pixel features, builds a multiscale feature modulation module, and performs recurrent depth refinement through a parameter-shared decoder at a fixed resolution. This network design enables our R-MSFM to maintain a more lightweight architecture and fundamentally avoid error propagation caused by the coarse-to-fine design. Furthermore, we introduce the mask geometry consistency loss to facilitate our R-MSFM for geometry consistent depth learning. This loss penalizes the inconsistency of the estimated depths between adjacent views within the nonoccluded and nonstationary regions. Experimental results demonstrate the superiority of our proposed R-MSFM both at model size and inference speed, and show state-of-the-art results on two datasets: KITTI and Make3D. |
Authors |
Zhongkai Zhou , Xinnan Fan , Pengfei Shi , Yuanxue Xin , Dongliang Duan 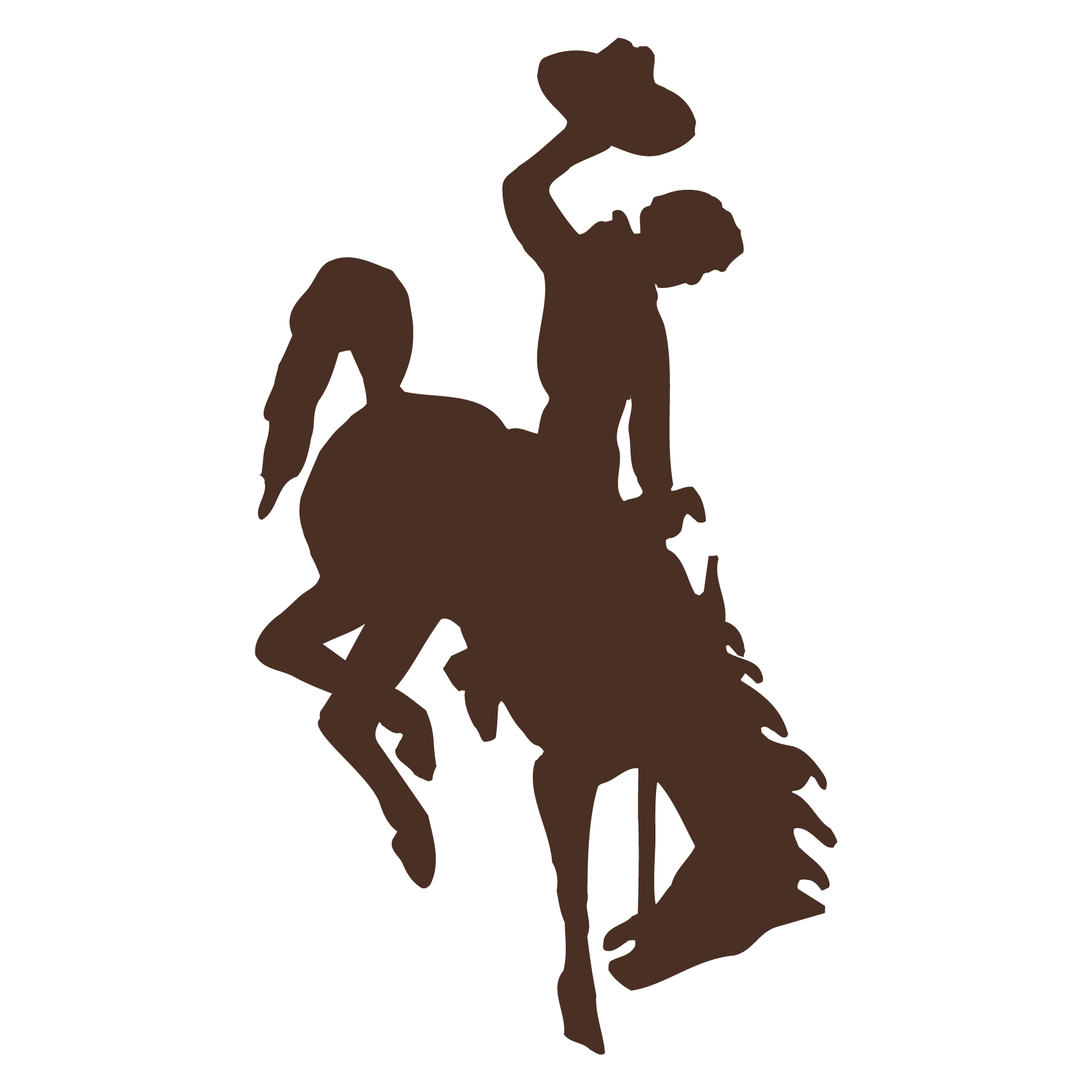 , Liuqing Yang
|
Journal Info |
IEEE Computer Society | IEEE Transactions on Pattern Analysis and Machine Intelligence , vol: 46
, iss: 12
, pages: 9551 - 9566
|
Publication Date |
6/27/2024 |
ISSN |
0162-8828 |
Type |
article |
Open Access |
closed
|
DOI |
https://doi.org/10.1109/tpami.2024.3420165 |
Keywords |
Feature (linguistics) (Score: 0.54216856) , Computational Geometry (Score: 0.42911488)
|