Abstract |
Leveraging machine learning algorithms (ML) for AC optimal power flow (ACOPF) is an inevitable tendency in power system analysis and operation. A wide range of ML models' applications such as deep neural networks and convolutional neural networks are utilized to tackle the ACOPF more efficiently. For large-scale power systems, the disadvantages of deep neural networks (DNNs) are alternated by manipulating weighted-convolution neural networks (WCNNs) to input a whopping number of the entire system's parameters for efficient training. However, the strengths of DNNs are undeniable, and they should be exploited to improve their efficacy and efficiency. By combining DNNs and WCNNs within a model, named an ensemble-weighted convolutional neural network (EWCNN), this paper proposes a new machine-learning model based on DNNs and WCNNs to increase the model fidelity and accuracy. The proposed approach is tested on three IEEE case studies to exemplify its capability. The empirical experiments show an improvement in reducing the bias-variance tradeoff commonly found in machine learning problems and raising the prediction accuracy. |
Authors |
Quan Tran 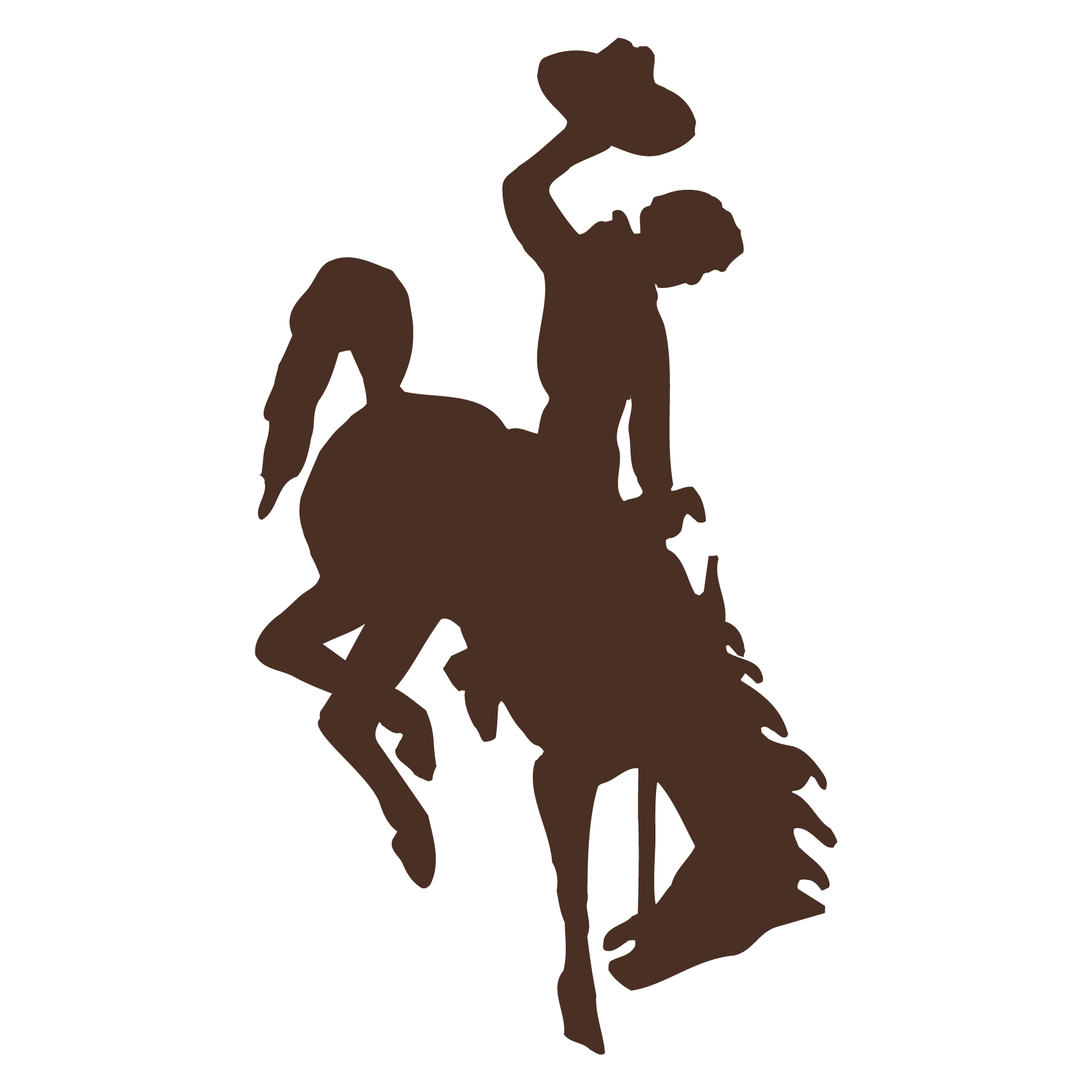 , Joydeep Mitra , Nga Nguyen 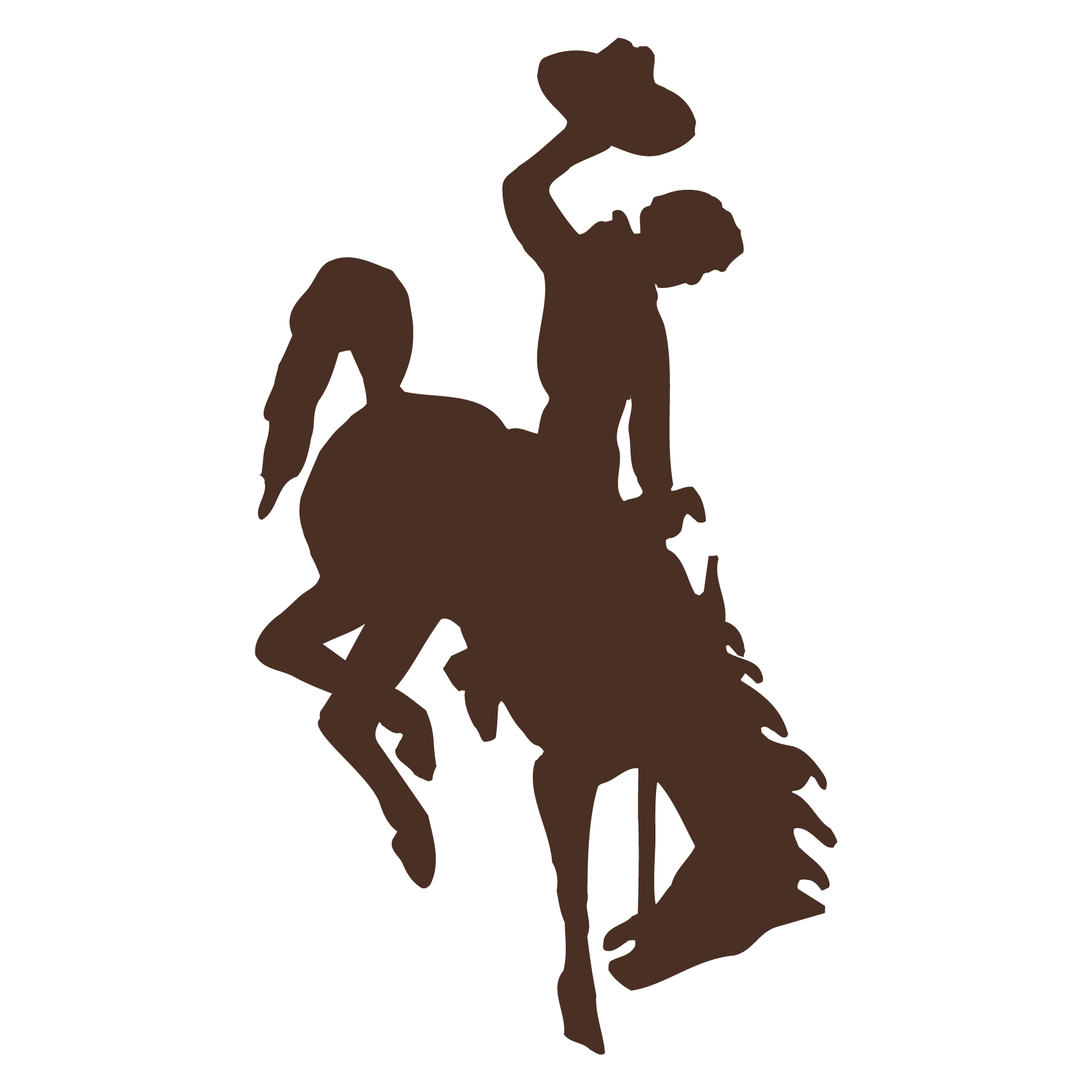
|
Journal Info |
Institute of Electrical and Electronics Engineers | 2025 IEEE Texas Power and Energy Conference (TPEC) , pages: 1 - 6
|
Publication Date |
3/6/2025 |
ISSN |
|
Type |
article |
Open Access |
closed
|
DOI |
https://doi.org/10.1109/tpec63981.2025.10906990 |
Keywords |
|