Abstract |
Accurate prediction of groundwater level (GWL) over a period of time is of great importance for groundwater resources management. Machine learning techniques due to their great performance have recently been used for this problem. Previous methods, however, did not consider the spatial relationships between wells due to the difficulty to handle unstructured well location data. In this paper, a graph neural network (GNN) is used to forecast groundwater dynamics where it can represent each well as a node in the graph. The spatial information is, thus, extracted from an interconnected network using graph convolution layers with a self-adaptive adjacency matrix. The temporal features of the sequence are obtained by gated temporal convolutional networks. The model was applied and evaluated for wells in the southwest area of British Colombia in Canada using data about 11 years (2010–2020). The proposed model performs better in terms of all the defined evaluation metrics, when compared with two baseline models: long short-term memory (LSTM) and gated recurrent units (GRU). Moreover, when the spatial dependencies are completely unknown, the model can still learn them from the data and obtain comparable performance. Furthermore, the proposed model has a high efficiency since it can simultaneously model GWL change for all monitoring wells in the system. We also demonstrated that the spatial dependencies between each well could be intuitively interpreted from the learned adjacency matrix. |
Authors |
Bangyi Tao 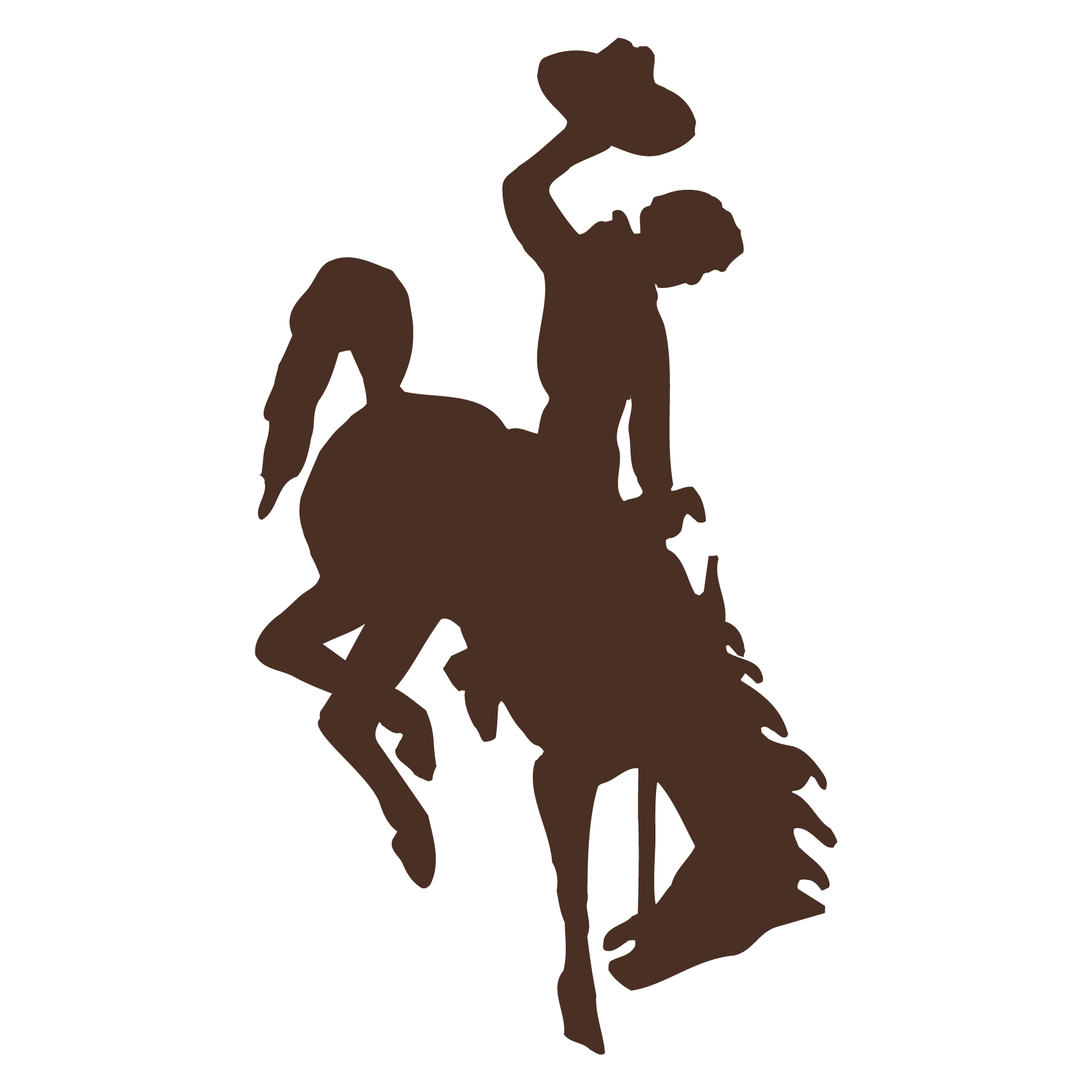 , Pejman Tahmasebi
|
Journal Info |
Elsevier BV | Journal of Hydrology , vol: 616
, pages: 128792 - 128792
|
Publication Date |
11/28/2022 |
ISSN |
0022-1694 |
Type |
article |
Open Access |
closed
|
DOI |
https://doi.org/10.1016/j.jhydrol.2022.128792 |
Keywords |
Groundwater Level Forecasting (Score: 0.677815) , Rainfall-Runoff Modeling (Score: 0.547475) , Watershed Simulation (Score: 0.539503) , Groundwater Flow (Score: 0.534833) , Hydrological Modeling (Score: 0.528633)
|