Abstract |
A robotic gym equipped with several rehabilitation robots enables multiple patients to engage in simultaneous exercise, all overseen by one therapist. The effectiveness of multipatient training could potentially be enhanced by dynamically allocating patients to specific robots based on continuously monitored patient data. This paper focuses on training an Inverse Reinforcement Learning agent for dynamic patient-robot task assignment in a multi-patient, multi-robot rehabilitation gym. The agent learns a reward model that comprehensively captures the intricacies of the expert's assignment strategy based on expert data, exhibiting improved generalization capabilities for unseen scenarios compared to our previous work using supervised learning. The output of the reward model estimates if the assignment follows the domain expert's underlying assignment strategy. The proposed approach was evaluated using a simulator designed to emulate the outcomes of patient exercises with robots. Our proposed method achieved similar performance to that of a domain expert and outperformed four baseline assignment methods. |
Authors |
Varun R. Bharadwaj , Benjamin A. Miller , Domen Novak , Chao Jiang 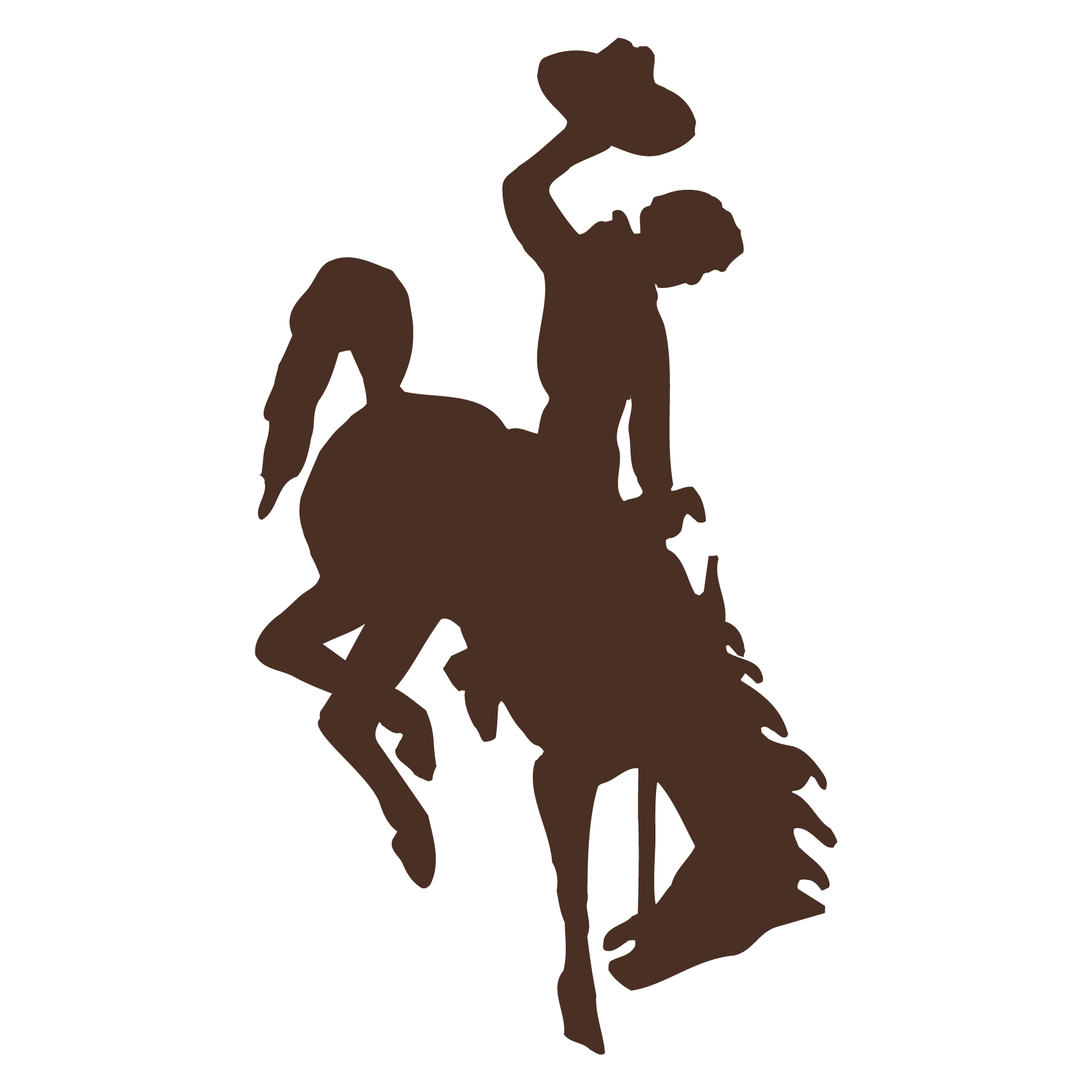
|
Journal Info |
Institute of Electrical and Electronics Engineers | 2022 9th IEEE RAS/EMBS International Conference for Biomedical Robotics and Biomechatronics (BioRob) , pages: 1815 - 1821
|
Publication Date |
9/1/2024 |
ISSN |
|
Type |
article |
Open Access |
closed
|
DOI |
https://doi.org/10.1109/biorob60516.2024.10719705 |
Keywords |
|