Abstract |
Current methods for solving optimal power flow have difficulties obtaining real-time solutions due to the complexity and nonlinearity of large power systems. Taking advantage of machine learning (ML) algorithms, trained machine-learning models are viable alternatives to tackle the challenges of online OPF-solving. This paper proposes the application of neural network models with self-attention to reduce an inductive bias and overcome the drawbacks of traditional ML-based approaches in solving OPF. By incorporating all power system parameters into a multiple-channel matrix akin to an image, the proposed weighing-convolutional neural network (WCNN) can extract typical features from training data and learn the underlying patterns. The high computational burden is alleviated through the training phase, and an OPF solution can be produced instantly by inference of the trained model. The proposed method is implemented on three IEEE standard systems to prove its efficacy. The experiment results show that the proposed WCNN algorithm obtains a high level of accuracy, which facilitates its potential capability for deployment in the practical operation of complex power systems. |
Authors |
Quan Tran 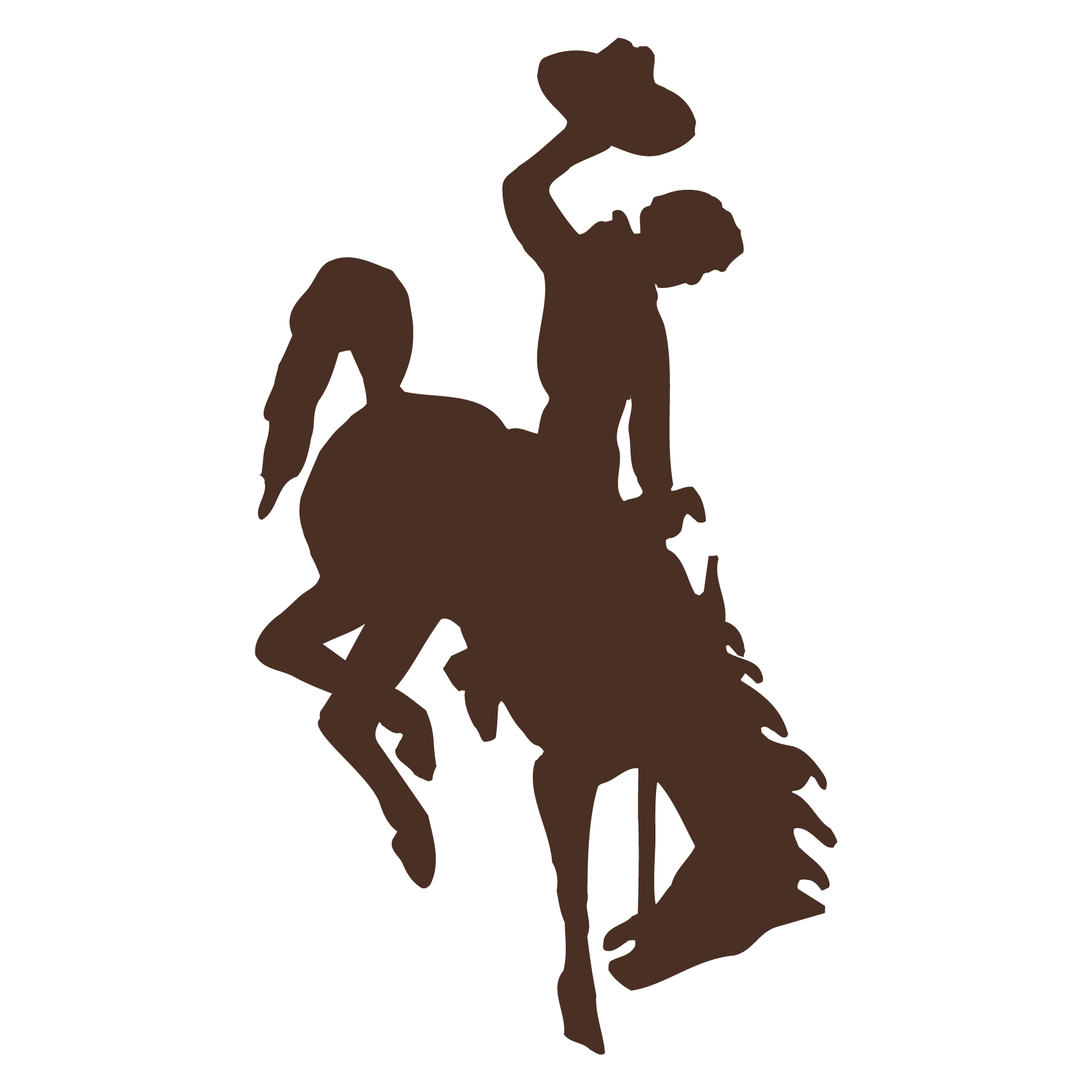 , Joydeep Mitra , Nga Nguyen 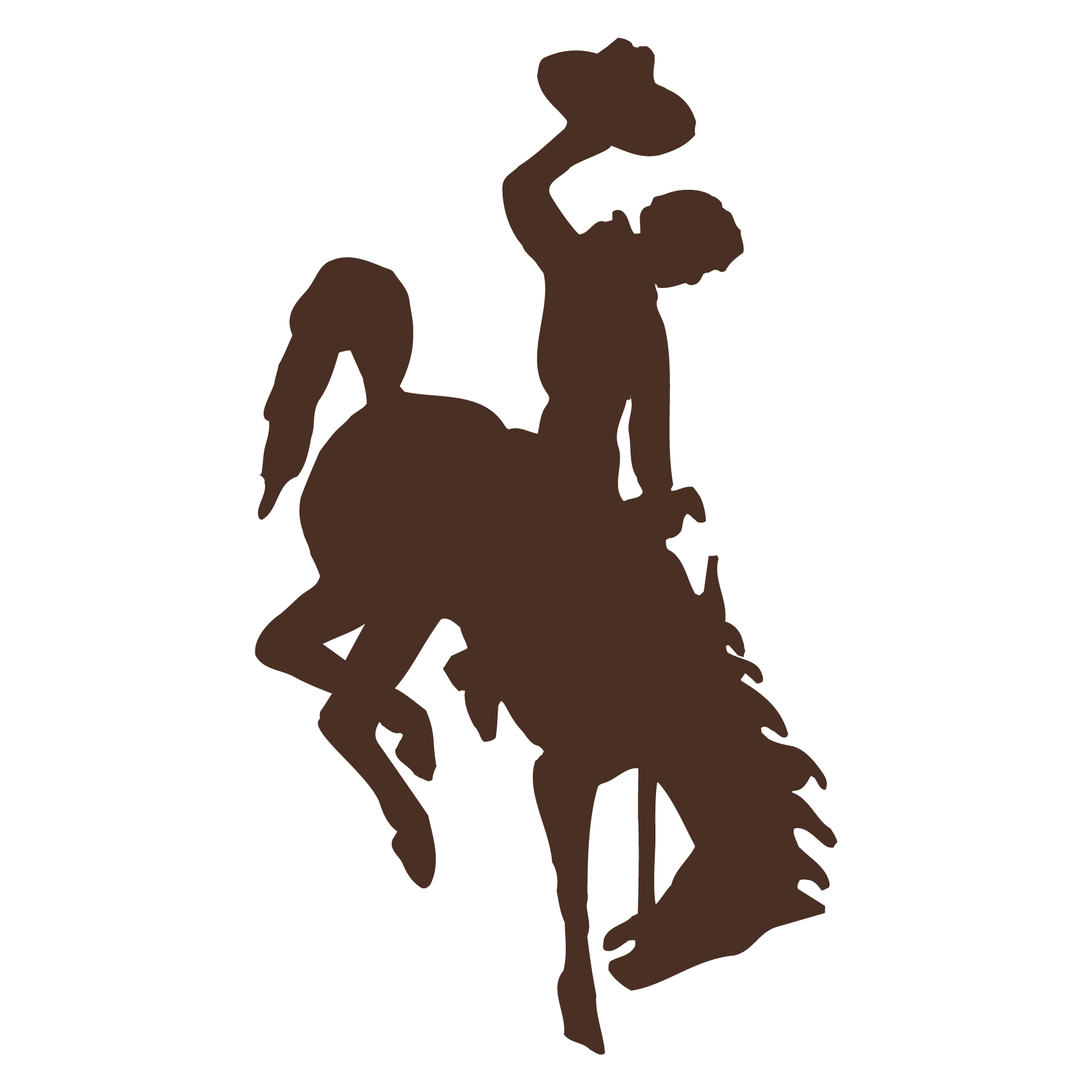
|
Journal Info |
Institute of Electrical and Electronics Engineers | 2024 22nd International Conference on Intelligent Systems Applications to Power Systems (ISAP) , pages: 1 - 7
|
Publication Date |
11/8/2024 |
ISSN |
|
Type |
article |
Open Access |
closed
|
DOI |
https://doi.org/10.1109/isap63260.2024.10744199 |
Keywords |
|