Abstract |
Most current hyperspectral image classification (HSIC) models require a large number of training samples, and when the sample size is small, the classification performance decreases. To address this issue, we propose an innovative model that combines an orthogonal capsule network with meta-reinforcement learning (OCN-MRL) for small sample HSIC. The OCN-MRL framework employs Meta-RL for feature selection and CapsNet for classification with a small data sample. The Meta-RL module through clustering, augmentation, and multiview techniques enables the model to adapt to new HSIC tasks with limited samples. Learning a meta-policy with a Q-learner generalizes across different tasks to effectively select discriminative features from the hyperspectral data. Integrating orthogonality into CapsNet reduces the network complexity while maintaining the ability to preserve spatial hierarchies and relationships in the data with a 3D convolution layer, suitably capturing complex patterns. Experimental results on four rich Chinese hyperspectral datasets demonstrate the OCN-MRL model’s competitiveness in both higher classification accuracy and less computational cost compared to existing CapsNet-based methods. |
Authors |
Prince Yaw Owusu Amoako , Guo Cao , Boshan Shi , Di Yang 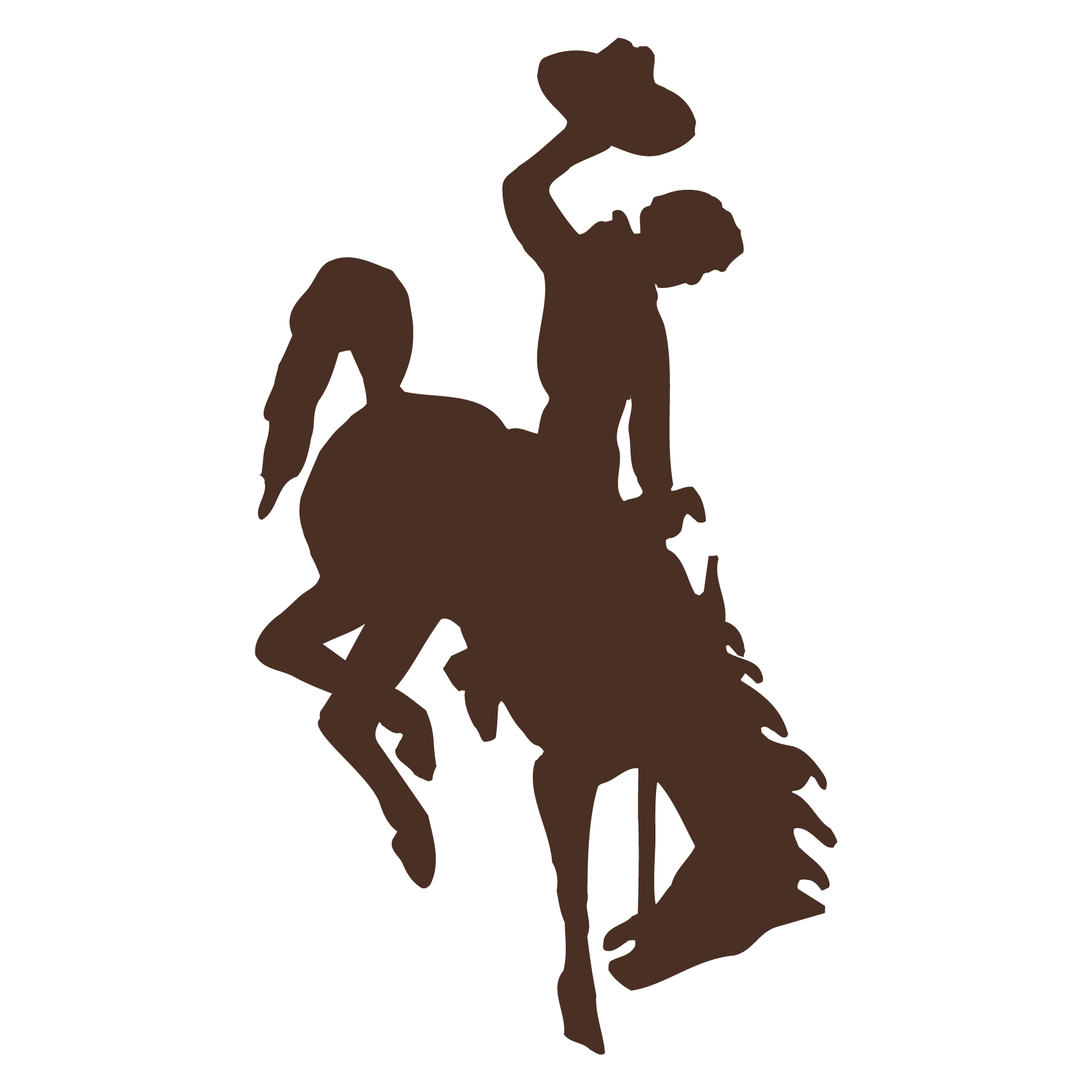 , Benedict Boakye Acka
|
Journal Info |
Multidisciplinary Digital Publishing Institute | Remote Sensing , vol: 17
, iss: 2
, pages: 215 - 215
|
Publication Date |
1/9/2025 |
ISSN |
2072-4292 |
Type |
article |
Open Access |
gold
|
DOI |
https://doi.org/10.3390/rs17020215 |
Keywords |
Sample (material) (Score: 0.5117023) , Capsule (Score: 0.45311463)
|