Abstract |
Summary Accurate subsurface geophysical characterization is crucial for the long-term safety and success of geologic carbon sequestration (GCS) projects. While traditional deterministic inversion methods are computationally efficient, they often fail to adequately reveal uncertainties in the inverted model. However, the information about model uncertainties is essential for informed decision-making and effective risk management in GCS projects. This study introduces the SVGD-AE method, a novel probabilistic inversion approach that estimates subsurface properties and quantifies uncertainties from geophysical data. The SVGD-AE method combines Stein Variational Gradient Descent (SVGD) for efficient sampling in high-dimensional spaces with an autoencoder (AE) neural network for model reparameterization, aiming to address computational challenges like gradient vanishing and gradient incompatibility between different reservoir parameters. We have applied the SVGD-AE method to the Illinois Basin - Decatur Project (IBDP), a large-scale CO2 storage project in Decatur, Illinois, USA. The resulting petrophysical models with quantified uncertainties provide valuable insights into subsurface properties, which is of great importance for feasibility analysis, informed decision-making, and ensuring the long-term safety of CO2 storage at the IBDP. |
Authors |
Mingliang Liu , Dario Grana 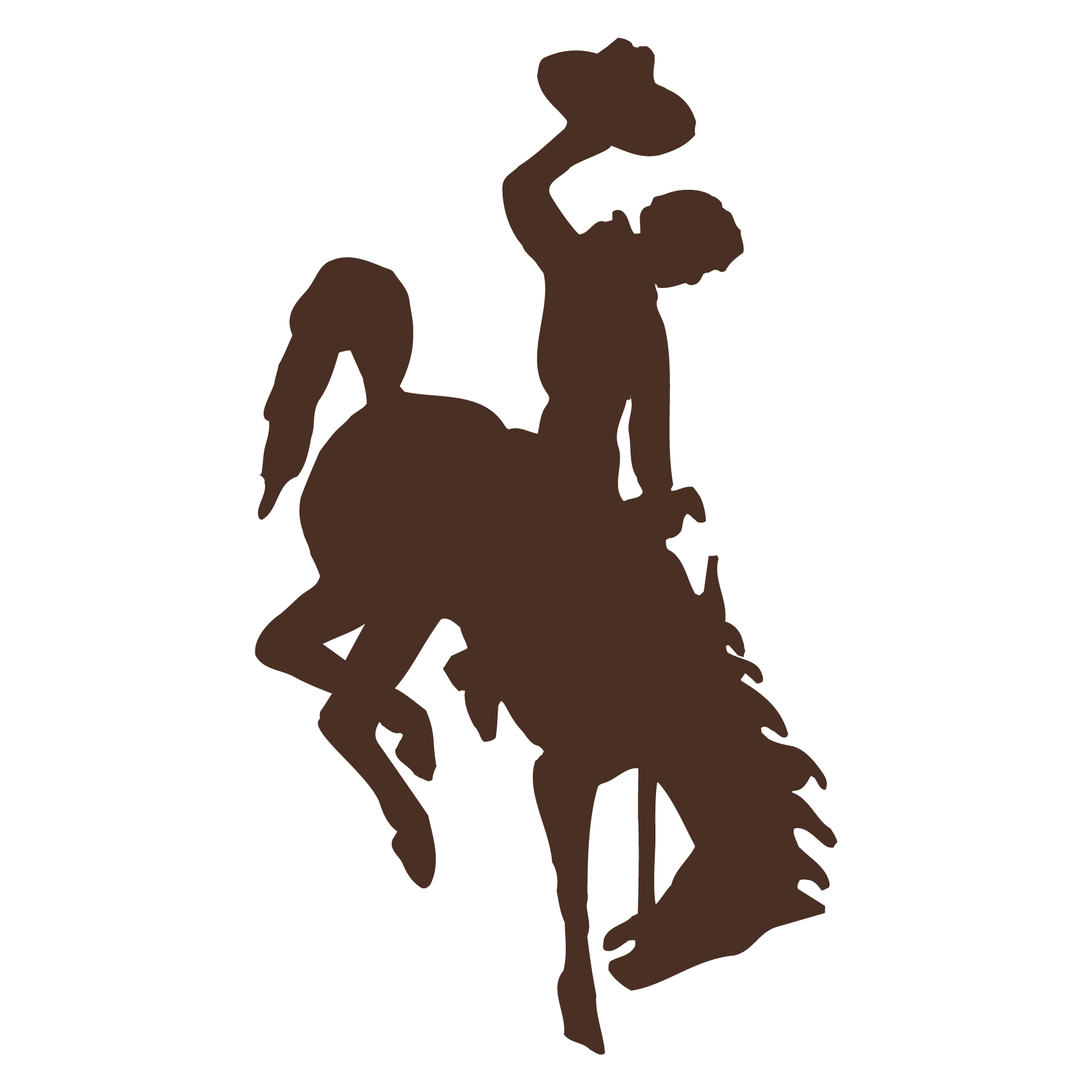 , Tapan Mukerji
|
Journal Info |
Not listed | 85th EAGE Annual Conference & Exhibition
|
Publication Date |
1/1/2024 |
ISSN |
Not listed |
Type |
article |
Open Access |
closed
|
DOI |
https://doi.org/10.3997/2214-4609.202410656 |
Keywords |
Geological Modeling (Score: 0.584565) , Geological Mapping (Score: 0.546423) , Geophysical Imaging (Score: 0.516941) , Hydrogeological Assessment (Score: 0.511343) , Seismic Data Processing (Score: 0.50975)
|