Abstract |
Deep reinforcement learning (deep RL) has the potential to replace classic robotic controllers.State-of-the-art Deep Reinforcement algorithms such as Proximal Policy Optimization, Twin Delayed Deep Deterministic Policy Gradient and Soft Actor-Critic Reinforcement Algorithms, to mention a few, have been investigated for training robots to walk.However, conflicting performance results of these algorithms have been reported in the literature.In this work, we present the performance analysis of the above three state-ofthe-art Deep Reinforcement algorithms for a constant velocity walking task on a quadruped.The performance is analyzed by simulating the walking task of a quadruped equipped with a range of sensors present on a physical quadruped robot.Simulations of the three algorithms across a range of sensor inputs and with domain randomization are performed.The strengths and weaknesses of each algorithm for the given task are discussed.We also identify a set of sensors that contribute to the best performance of each Deep Reinforcement algorithm. |
Authors |
James Mock , Suresh Muknahallipatna 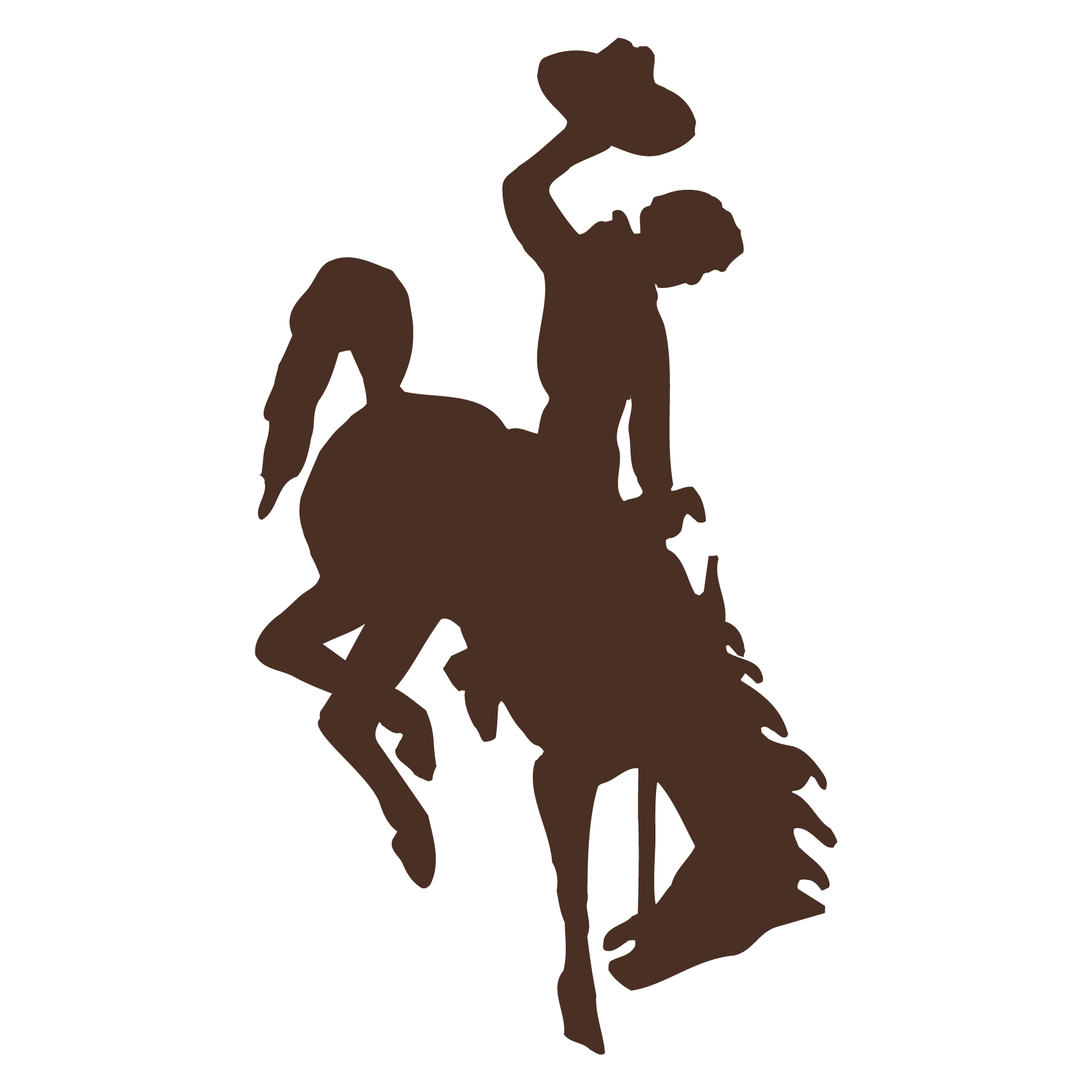
|
Journal Info |
Scientific Research Publishing | Journal of Intelligent Learning Systems and Applications , vol: 15
, iss: 01
, pages: 36 - 56
|
Publication Date |
1/1/2023 |
ISSN |
2150-8402 |
Type |
article |
Open Access |
gold
|
DOI |
https://doi.org/10.4236/jilsa.2023.151003 |
Keywords |
Passive-Dynamic Walkers (Score: 0.601222) , Dynamic Walking (Score: 0.594058) , Quadruped Robots (Score: 0.551871) , Robotics (Score: 0.511718) , Legged Robots (Score: 0.508614)
|